Blogs
Xplore_AI: What’s in a name? Be specific!
Written by: Chris Stephenson
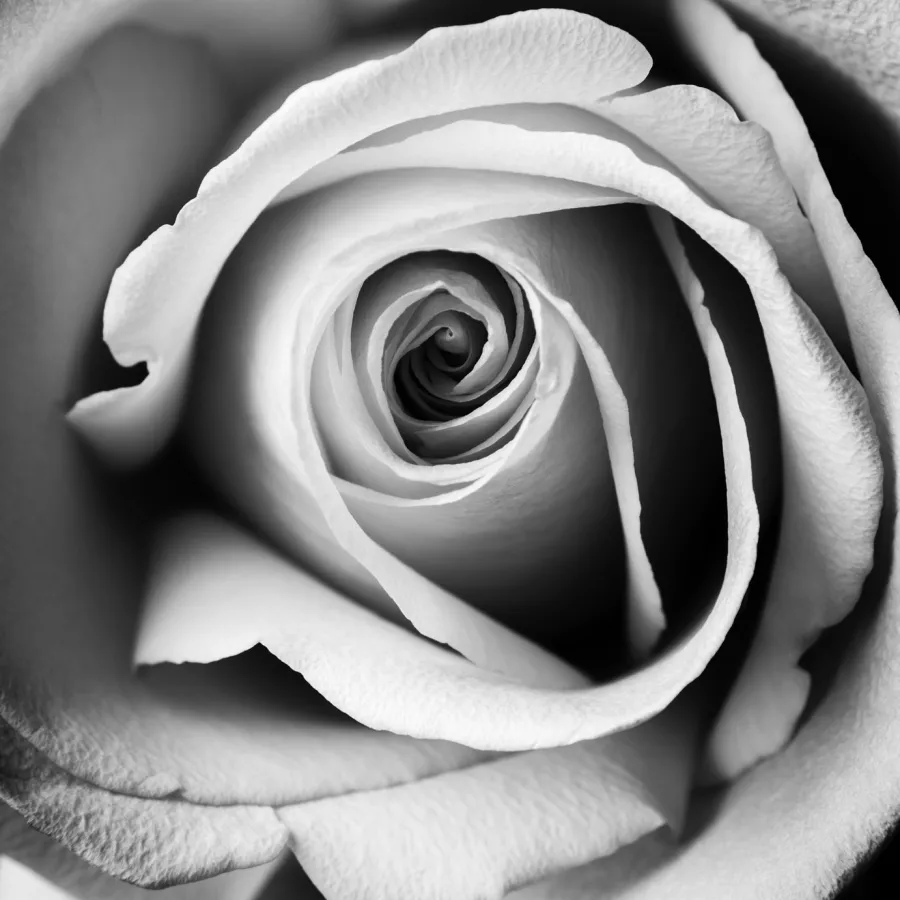
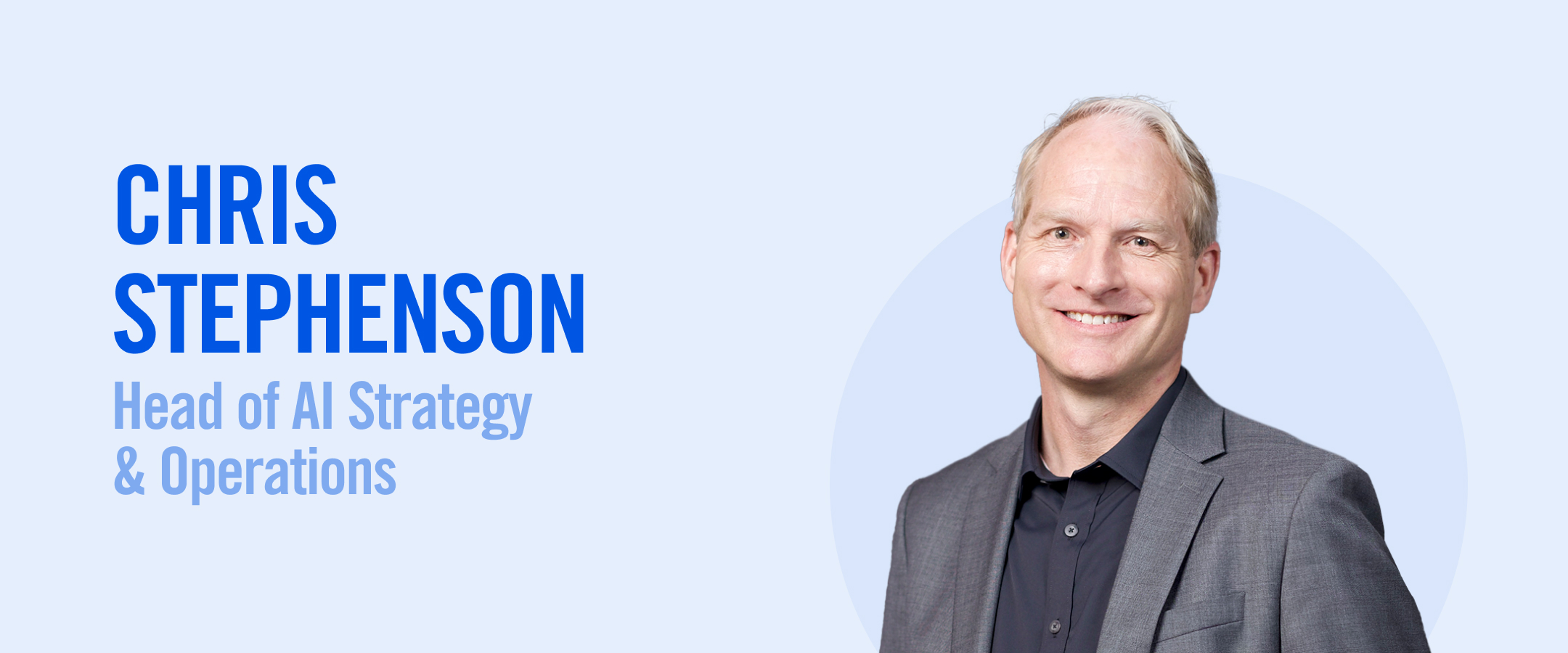
XPLORE_AI: What’s in a name? Be specific!
“What's in a name? That which we call a rose, by any other name would smell as sweet.” In this one famous line from Romeo and Juliet, Juliet’s heart-felt reasoning seems as poignant as it is poetic. However, naming things is a fundamental aspect of human communication, helping us make sense of a complex world. And at Nuix, data labeling plays a critical role in our AI-driven solutions, exemplifying one of our Responsible AI (RAI) principles, Specificity.
Can you be more Specific?
It may not make great literature, but adherence to the Specificity principle puts our customers at center stage, empowering them with the ability to ensure their AI models are well understood, operating properly, and in alignment with expected outcomes. In Nuix parlance, Specificity simply means that the AI tools are—or can easily be specialized to be—fit-for-purpose, which is critical to operating ethical, trustworthy, and defensible AI. One of the foundational capabilities that makes this possible is a proprietary, no-code approach that is unique to Nuix.
What’s in a label? A lot more than you might think!
In machine learning (ML), data labeling involves tagging digital assets (like images, text, or videos) with descriptive labels (like "gun," "child," or "patent"). This helps the computer learn to recognize and classify new, similar data correctly in the future. Essentially, labeled data acts as a guide, teaching the machine to make accurate predictions or decisions when new data is analyzed. This is an important part of building specific, purposeful machine learning models.
Simple, right? Well, not so fast. Data labeling is just one part of a broader data preparation process (including data collection, cleaning, normalizing, etc.). And of course, all of this is a precursor to building and optimizing performant ML models. Suffice to say that these highly manual processes typically chew up at least 80% of the time spent on most ML projects using traditional methods. And this is for experienced data scientists and engineers!
Fortunately, for our customers, the above steps can be achieved in a few clicks or eliminated altogether, because Nuix software comes standard with hundreds of pre-built models. And if we don’t have what you need out of the box, existing models can be adjusted—or new specialized models can be built—in minutes using a no-code model editor. This means subject matter experts can skip the data prep and model building phases and jump right to the ‘get actionable insights’ phase. The proper label for that phase is Inference, which is another core strength of our differentiated AI-powered solutions and will be covered in more depth in next month’s post.
AI Your Way
As one of our core RAI principles, Specificity is much more than an empty platitude listed on our web site, but demonstrable within the solutions we build. It ensures that our customers have the flexibility and control they need to optimize both the performance of their data analysis workflows and the defensibility of the outputs. And our customers are experiencing this firsthand, particularly those with a range of use cases or rapidly changing requirements such as:
- A global advisory focused on data breach response
- A governmental agency focused on redundant, obsolete, trivial (ROT) data remediation
- A top 10 pharmaceutical company focused on both investigations of IP leakage and analysis of research data to drive new drug discover and decisioning.
- A tax authority combating fraud.
Benefits of Specificity
Some of the key benefits of embracing Specificity include:
- Improved Accuracy: Using specific features relevant to the task enhances accuracy and reduces errors. By selecting or building models that are particularly well-suited to the problem can lead to better performance.
- Relevance: Specific models can focus on relevant data, reducing the risk of learning noise and overfitting. Additionally, models tailored to the specific task are likely to generalize better to new, unseen data within the same context.
- Efficiency: Specific models can be optimized to run efficiently, focusing on relevant data and features, which uses less computational power and memory and speeds up the training process and analysis times.
- Interpretability: Specific models produce more interpretable results, as they are built with a clear understanding of the task and data. They also tend to generate insights that are directly applicable and actionable for the problem.
- Regulatory Compliance: Specific models can be designed to comply with industry regulations and standards, ensuring legal and ethical use of AI. This includes enhanced traceability and auditability of the model's decisions, which is crucial for compliance.
Summary
Specificity is a critical aspect of our RAI strategy, enabling organizations to develop and leverage more effective, efficient, and reliable models that deliver high value and meet the precise needs of their applications and evolving use cases. In a world where it can often feel like we’re ceding too much control to AI, Specificity gives our customers clarity (via interpretable insights), confidence (via improved accuracy and defensibility), and a competitive edge (via automation and time-to-value).
Ps. Just to show that I’m balanced and fair minded, I’ll give Juliet the final word on this…but through a modern teenage lens.
Hey Romeo! 🥰 Just checked out Nuix Neo’s AI tech and OMG, it's like totally lit! 🔥✨ Super explainable and trustworthy AF. It's like having Friar Lawrence spill all the tea but without any drama. 🤫🔍 No cap, you gotta see it to believe it. #TechGoals #TrustworthyAI #NoDrama
xoxo💌💕